In an era where data drives the world, it is not a surprise that agriculture, too, is embracing the immense possibilities of data-driven decision-making through smart farming and agri-environmental monitoring. In this context, AgriDataValue project has been born to enhance smart-farming capacities, competitiveness, and fair income by introducing a fully distributed platform of platforms based on cutting-edge technology, such as complex deep learning-based decision support systems to revolutionize the food supply chain.
As the storage and computing capabilities go forward, deep learning, generative models, and AI in general have taken the spotlight. However, in the agriculture domain, there are several challenges that complicate training these AI models.
1. The Data Dilemma: Despite the increase in agri-environmental monitoring, in several use cases the scarcity of data harms the generalization capabilities of the models.
2. Representation Reality: In instances where data is accessible, it often lacks in representing the diverse realities of agriculture: geographic location, crop types and farming practices introduce biases that hinder the effectiveness of AI solutions.
3. Privacy Predicament: The presence of sensitive and private data makes entities reluctant to share their insights with other entities.
4. Non-technical end users: In agriculture, the end-users are often farmers or technicians with limited model interpretation experience. This poses a challenge as complex AI models often operate as black boxes, requiring enhanced efforts for comprehension and trust-building.
AgriDataValue’s Game-Changing Solution
How will AgriDataValue tackle these challenges?
Federated Learning (FL) is a Machine Learning approach that prioritizes privacy and decentralization. It enables models to be trained on data distributed across multiple devices without the need of sharing any sensitive information. Following this paradigm, devices or clients train their AI models with their local data and share their model updates with a central sever that aggregates them. This places FL at the forefront of innovation across diverse privacy-sensitive fields, such as medicine, finances, and agriculture.
In this context, AgriDataValue will go one step further and deploy a Hierarchical Federated Learning (HFL) approach that optimizes the resource usage by introducing a structured hierarchy into the FL process. This hierarchical arrangement allows for multi-level communications, making it a highly scalable solution and adaptable to domains like agriculture, with a high variety of data sources. The hierarchy is composed by a root server, that generates the global model, the end-users or edge devices, and several intermediate layers of servers. These intermediate server layers aggregate the model updates coming from lower layers and send the aggregated models to other aggregators positioned above in the hierarchy. After the final aggregation performed by the root server, the global model is forwarded down to the hierarchy layers.
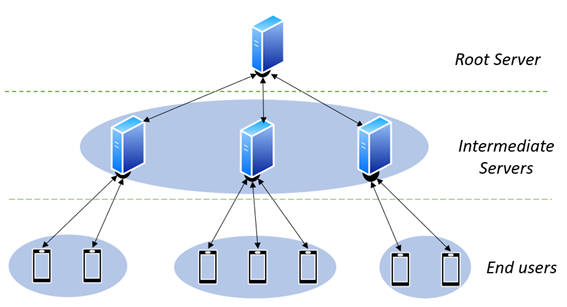
In this way, AgriDatavalues solution proposes the fusion of HFL with privacy-preserving algorithms and explainable AI (XAI), to overcome the challenges arising when constructing reliable decision systems rooted in trustworthy and comprehensible AI. In doing so, AgriDataValue platform of platforms aspires to generate AI models from diverse data sources, all while efficiently safeguarding the privacy of sensitive information. These solutions position themselves as a pioneering force in driving innovation and research within the agricultural sector.