Agriculture is once again going through a critical moment. In addition to the historical challenge of having to feed a growing population adequately, the need to anticipate the challenges posed by climate change is now becoming more pressing, given the early materialization of the risks that scientists had been anticipating. To this is added the need to modify agricultural practices in such a way that the quantity and quality of agricultural production is maintained (and increased), while reducing the impact on the environment in general and on the soil in particular, and, in the same way, that agricultural holdings are economically sustainable.
One of the agricultural tasks that has the most environmental and economic impact is the application of phytosanitary products for the control of pests, whether they are fungi or insects. Reducing the application to the cases and moments in which they are strictly necessary improves the sustainability of agricultural holdings. Achieving this improvement requires that treatments be applied at the times, phenological states, in which they are really going to be effective and, only if, there is a real risk of infection or plant damage.
During the last 300 years, models of predicting the phenological evolution of plants and the risk of diseases have been developed. These models are based on the linking of field observations, of the phenological states or of the presence of pests, with meteorological data, fundamentally temperature and environmental humidity. These models can be calibrated to adjust to the characteristics of a particular space, for which it is necessary to have precise meteorological observations and, above all, field observations, which makes their application by farmers in general difficult.
In recent years, taking advantage of the capabilities of data capture, processing, and visualization that current technologies offer, more advanced models of predicting phenology and disease risk have been developed. Developed by multidisciplinary teams, they take advantage of diverse data sources to make precise predictions, at the level of a plot, while also being general and applicable to different geographical regions. Many of these models apply Big Data and Artificial Intelligence technologies to process data from weather stations, weather forecasts, multispectral satellite images (Sentinel 2), or, among other data sources, from field observations. However, most of these models are still limited to specific geographical areas. One of the reasons for this limitation is the lack of datasets of field observations.
It is not a question of the absence of datasets of field observations. The problem is that there is a reluctance to share them for use. This reluctance would probably disappear if there was confidence in the use of the data that is going to be carried out. Data spaces, such as the one being developed in the Agridatavalue project, have as one of their objectives to overcome this reluctance by guaranteeing the ownership and sovereignty of the data shared in them: the control of their use. They also allow shared use in the cloud and access to resources that allow the massive processing of data.
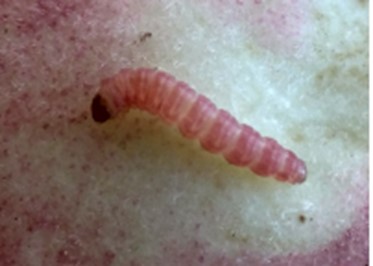
The use case UC 3.1: Fruit trees disease forecast/detection developed by SARGA and ITAINNOVA will take advantage of the capabilities of the Agridatavalue data space and the resources deployed by several partners of the project to develop models of predicting phenology and risk of diseases in fruit trees (apple, pear, peach, etc.) from plots located in Aragon. To do this, it will use meteorological data from networks of stations managed by SARGA, weather forecasts, and Sentinel 2 multispectral images. However, the most appreciated resource will be the field observations, of the phenological evolution of fruit trees and the presence of pests, from the FARA network managed by the Government of Aragon.
The development of these models will allow farmers to optimize the use of phytosanitary products, reducing their environmental impact and improving the economic sustainability of their holdings. It will also allow them to make better decisions about the timing of harvests, which will improve the quality and marketability of their products.