The agricultural sector is facing significant challenges in managing and exchanging data across diverse systems and stakeholders. With the proliferation of sensors, weather data, farming machinery, and other digital tools, there is an increasing need for standardized methods to ensure that agricultural data can be exchanged seamlessly and with a clear, consistent meaning. The AgriDataValue project is at the forefront of tackling this issue, focusing on developing data models and enhancing semantic interoperability within the agriculture domain.
Data Models: The Backbone of Standardized Agricultural Data
In the context of the AgriDataValue project, data models play a crucial role. These models provide a structured and standardized way of organizing agricultural data, making it easier for stakeholders to understand, share, and use. By establishing clear frameworks for data representation, AgriDataValue helps ensure that agricultural data from various sources—ranging from IoT sensors and weather stations to farm management systems—can be efficiently integrated and analyzed (W3C, 2014).
The AgriDataValue platform serves as the central hub in the ecosystem, facilitating seamless data exchange and interoperability between various components. The diagram below illustrates the flow of data and interactions between the AgriDataValue platform and external data sources, such as IoT sensors, weather stations, and farm management systems, as well as the integration of standards like IDS, GAIA-X, AIM, and FIWARE. (Figure 1)
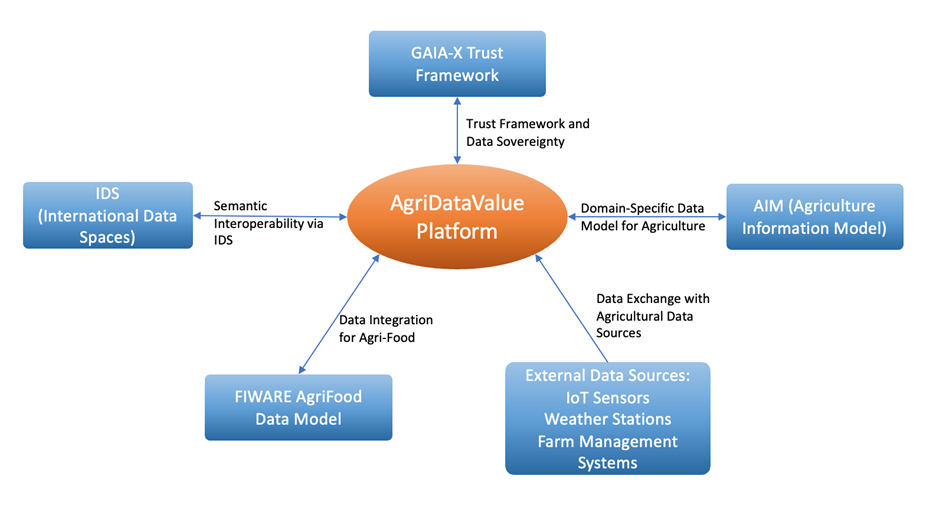
Semantic Interoperability: Ensuring Consistent Data Meaning
However, it is not enough just to organize data; we also need to ensure that the meaning of the data is preserved and understood across different systems and platforms. This is where semantic interoperability comes into play. Semantic interoperability ensures that data exchanged between different systems is not only compatible but also retains its intended meaning (International Data Spaces Association, 2020).
AgriDataValue achieves this by leveraging the International Data Spaces (IDS) standard and the GAIA-X Trust Framework, both of which provide robust guidelines for data exchange in the agriculture sector (GAIA-X, 2021). By aligning with the Agriculture Information Model (AIM) from the DEMETER project, AgriDataValue strengthens the way data is represented and exchanged within the sector (DEMETER Project, 2020).
The IDS framework and its reference architecture, IDS-RAM, facilitate the integration of various systems and ensure that data can be exchanged without ambiguity. AgriDataValue adheres to the IDS-RAM, which offers a comprehensive view of data structures, concepts, and vocabularies, allowing for consistent semantic interoperability across different platforms (International Data Spaces Association, 2020).
Integrating IDS and AIM for Sector-Specific Solutions
One of the key innovations of the AgriDataValue project is the integration of IDS Information Model and the Agriculture Information Model (AIM). The IDS model is domain-agnostic, providing a general framework that can be applied to a wide range of industries, while AIM is specifically designed to address the unique needs of the agriculture sector (DEMETER Project, 2020).
This integration allows AgriDataValue to provide both broad industry-wide data structures and domain-specific agricultural data elements. For example, while the IDS Information Model includes concepts such as data assets, contracts, and participants, the AIM brings in agricultural-specific elements like AgriParcel, Crop, Intervention, and Pest (W3C, 2012). This combination ensures that AgriDataValue can cater to the specific needs of the agricultural industry while remaining adaptable to cross-industry applications (FIWARE Foundation, 2020).
The Agriculture Information Model (AIM), developed under the DEMETER project, is a comprehensive framework designed to facilitate semantic interoperability in agriculture. The model is publicly accessible and available under the Creative Commons Attribution 4.0 License, which promotes open and transparent data exchange (DEMETER Project, 2020). AIM is structured into several modules, each addressing specific agricultural domains like crop management, animal husbandry, and intervention systems (W3C, 2014).
These modules are represented in standard OWL (Ontology Web Language) and RDF (Resource Description Framework) formats, ensuring compatibility with other data models and facilitating integration with various systems across the agriculture sector (W3C, 2012).
Enhancing Data Sovereignty and Security with GAIA-X
Another key element of the AgriDataValue project is its commitment to data sovereignty, security, and privacy. The GAIA-X Trust Framework plays a central role in this regard. By adhering to GAIA-X’s technical and organizational guidelines, AgriDataValue ensures that agricultural data is handled securely, preserving user privacy and complying with data protection regulations (GAIA-X, 2021).
This focus on trust and data security is essential for fostering confidence among data providers and consumers in the AgriDataValue ecosystem. The project implements mechanisms such as data anonymization and access control, ensuring that sensitive agricultural data is protected while still enabling meaningful insights and analysis (International Data Spaces Association, 2020).
The Road Ahead: Extending and Evolving the AgriDataValue Model
As the AgriDataValue project progresses, it remains focused on creating a flexible, extensible data model. This model is designed to evolve as new technologies, data sources, and user needs emerge within the agricultural sector (FIWARE Foundation, 2020).
In the coming stages, AgriDataValue plans to expand its semantic interoperability mechanisms by integrating other data models and frameworks. These include FIWARE AgriFood Data Model, which further enhances interoperability in the agri-food sector, and other industry-specific vocabularies that will continue to improve the integration of agricultural data (DEMETER Project, 2020).
The integration of such models will ensure that AgriDataValue can support the dynamic, evolving nature of agriculture and continue to provide value to stakeholders in the sector. Whether it’s enhancing precision farming, improving resource management, or supporting decision-making at the policy level, AgriDataValue is well-positioned to drive the future of agricultural data interoperability (Atzori et al., 2017).
The diagram below illustrates the flow of data from external sources such as IoT sensors, weather stations, and farm management systems, through data transformation and standardization, to the AgriDataValue platform, and finally to end-users like farmers and policymakers. This process ensures that agricultural data is standardized and integrated for actionable insights and effective decision-making. (Figure 2)

Conclusion
The AgriDataValue project stands as a key initiative in promoting data interoperability in the agricultural sector. By combining robust data models, semantic interoperability frameworks, and a commitment to data security, AgriDataValue is helping to pave the way for more efficient, transparent, and data-driven agricultural practices. As the project continues to grow, its impact on the sector will only become more profound, enabling farmers, policymakers, and businesses to make informed, data-driven decisions for a sustainable future (Stojanovic & Milinkovic, 2019).
References
1) DEMETER Project. (2020). Agriculture Information Model (AIM) Overview. Retrieved from https://www.demeter-h2020.eu
2) GAIA-X. (2021). GAIA-X Trust Framework. Retrieved from https://www.gaia-x.eu
3) FIWARE Foundation. (2020). FIWARE AgriFood Data Model. Retrieved from https://www.fiware.org
4) W3C. (2014). RDF 1.1 Primer. W3C Recommendation. Retrieved from https://www.w3.org/TR/rdf11-primer/
5) W3C. (2012). OWL 2 Web Ontology Language Document Overview. W3C Recommendation. Retrieved from https://www.w3.org/TR/owl2-overview/
6) Atzori, L., Iera, A., & Morabito, G. (2017). The Internet of Things: A survey. Computer Networks, 54(15), 2787-2805. https://doi.org/10.1016/j.comnet.2010.05.009
7) Stojanovic, J., & Milinkovic, D. (2019). Standards and Frameworks for Agricultural Data Integration. Sensors, 19(2), 289. https://doi.org/10.3390/s19020289
8) International Data Spaces Association. (2020). International Data Spaces Reference Architecture Model (IDS-RAM). Retrieved from https://www.internationaldataspaces.org