We are pleased to announce that the flagship project AgriDataValue has officially started. The project kick-off meeting will be held in Athens on the 22nd and 23rd of February 2023.
AgriDataValue will lead the pan-European digital transformation in agriculture. The project stands out for its technological superiority, scale of deployment, and comprehensive end-to-end multi-actor involvement aiming to contribute to the solution of global food needs, sustainable and efficient farming, and protection of the environment, by optimizing agricultural production via knowledge. Targeting the new Common Agricultural Policy (CAP) implementation, the project will drive developments in Precision Farming and Agri-Environmental monitoring and strengthen the Agricultural Digital Transformation at European Level.
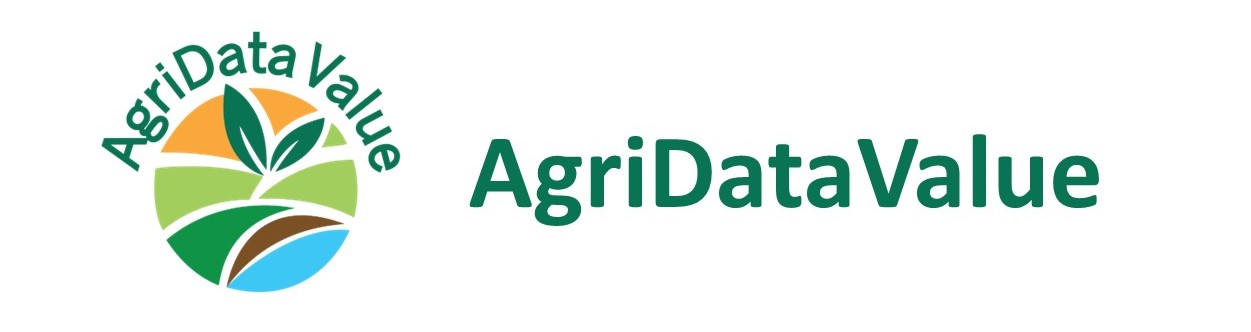
During the project’s lifetime, 23 pilots in 9 EU countries will be conducted, representing cultivated land of more than 181,000ha and livestock of more than 2,000 animals. In addition, more than 4,500 IoT sensors to measure (real-time) data will be installed. Within the project pilot activities, the technological superiority and functional flexibility of Synelixis’ SynField© and SynAir© product families, will be utilized. Synelixis is the coordinator of the consortium which consists of 30 partners from Greece, Spain, France, the United Kingdom, Luxembourg, Romania, Slovenia, Italy, Germany, Belgium, Poland, the Netherlands, Latvia, and Lithuania.